Software Development
What's the problem? Why use case beats tooling in driving AI value
24 June 2024 • 7 min read
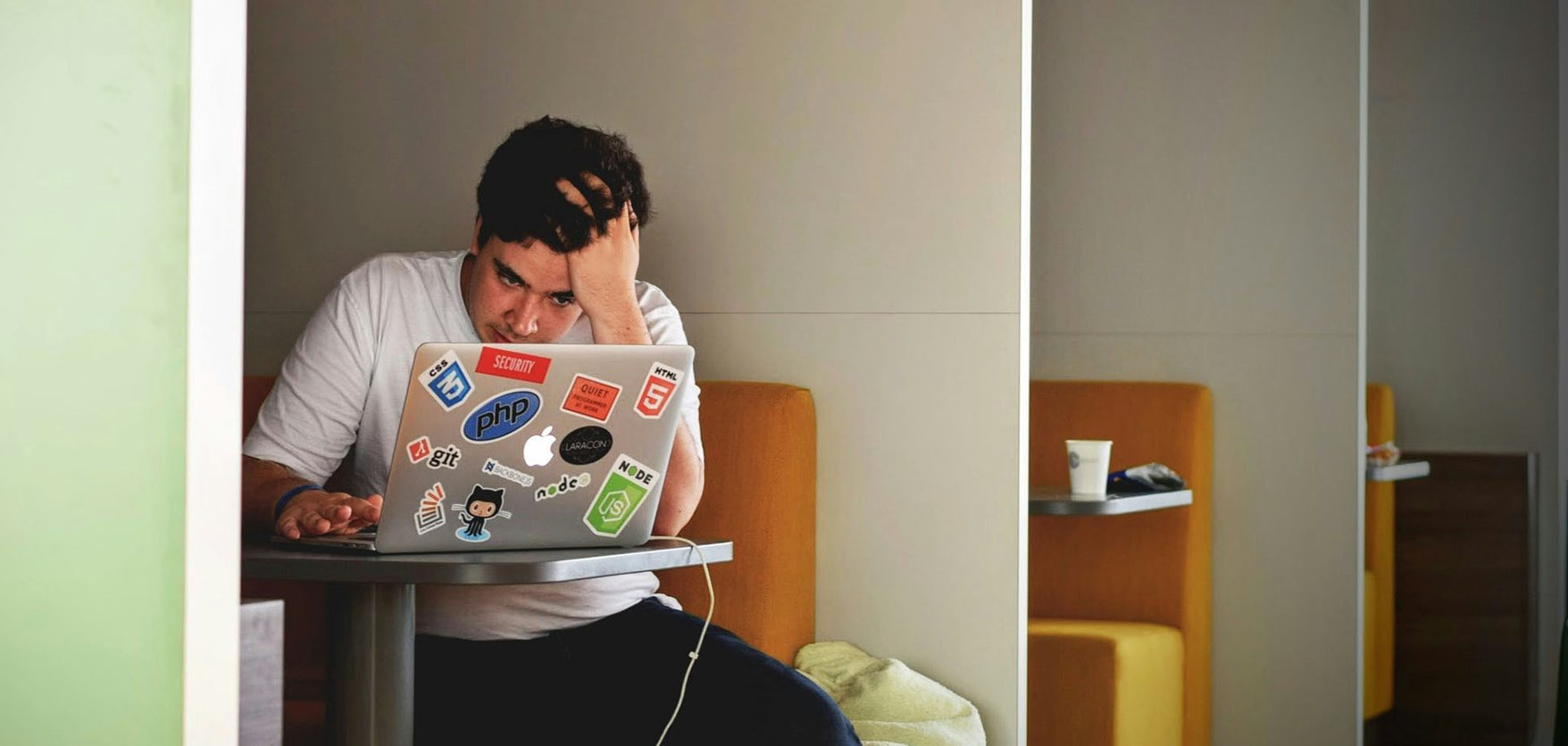
AI adoption has rapidly become the norm for businesses of all shapes and sizes, reflecting the hype that technologies like Generative AI (GenAI) have received, as well as people’s inevitable desire not to miss out on the next big thing. This raises the question, are businesses making informed decisions around AI adoption that will positively impact the top and bottom line, or are they moving too quickly because of a fear of missing out?
Measurable value should always be the end goal from any technology adoption, and AI is no exception. Concerningly, our research revealed that 44% of business leaders feel their management team is grossly underprepared to adopt AI.
That’s why in this blog, we’re taking a look at defining your organisational problems effectively and using that information to build AI use cases that will have a demonstrable impact on your business. Read on to ensure you get AI right, and accelerate value creation.
Start by recognising the strengths of AI
What is AI good at doing?
During the AI hype cycle it’s been presented as a panacea, a solution to all manner of problems. However, the truth is that there are some areas where AI truly excels - and can outperform a human - and there are others where human intervention is definitely still required. To see value quickly, it’s wise to focus on AI’s strengths and consider how they can be applied to your organisational context:
- AI is great at doing boring tasks like data tagging, metadata tagging, and pattern recognition. Due to the boring nature of these tasks, there’s a higher propensity for error when humans are doing them, it also takes people a lot longer
- AI is excellent at processing large amounts of data and providing insights quickly, it can free people up to focus their efforts on higher value activities
- AI is fantastic at identifying patterns, applying it in the right context can yield insights and opportunities that may not have been possible otherwise
Consider your business challenges
Now you have an understanding of what AI does well, you can consider the challenges that your business is facing. A strong use case is built on the alignment of technological capabilities and genuine organisational needs. After all, you don’t want to end up with a “solution in search of a problem”.
5 key factors to help you identify the right use cases for AI:
(1) Identifying the right problem:
The first step is understanding what problems or opportunities exist in your organisation that AI could address. These could range from customer service enhancements, recommendation engines, machine learning models that predict outcomes or simulate real world scenarios.
(2) Feasibility and impact:
Once a potential use case is identified, it’s essential to evaluate its feasibility and potential impact. This involves asking questions like: Can the problem be clearly defined? Is relevant data available to train AI models? What is the expected ROI? This helps in prioritising use cases that offer the most value.
(3) Integration and scalability:
Consider how the proposed AI solution will integrate with your existing systems. It’s important that the solution can scale and adapt as your business grows and changes. If an AI tool cannot integrate well with existing processes - or is too rigid - it might end up creating more problems than it solves.
(4) Ethical and legal considerations:
AI deployments must also be reviewed thoroughly to identify any ethical or legal implications, especially when it comes to data usage and privacy. Ensuring compliance with regulations like GDPR is crucial in maintaining trust and integrity in the use of AI.
(5) Continuous learning and adaptation:
Finally, successful AI implementation is not a one-time project but a continuous journey. AI systems need to be continuously trained and updated to adapt to new data and changing business environments. You need to be prepared to invest in ongoing training and development of your AI systems, and ensure your people have the skills necessary to drive the most value from them.
What does a good AI use case look like?
The validity of AI is bound to vary across industries and organisations, that’s why it’s critical to build a robust use case using the factors we’ve outlined above. If you’re looking for some inspiration, here’s some best practice examples to guide you:
- IAG Loyalty used intelligent AI recommendations to boost their customer reward redemptions, with 83% of users preferring the new AI-powered solution to a traditional search engine
- Evri created an AI-powered chatbot to improve the onboarding experience for seasonal couriers, demonstrably boosting retention and reducing support requests
- A leading biotechnology company used artificial intelligence and machine learning (AI/ML) to build a marketing recommendation engine that increased engagement by 200%
How do you measure the return on investment from your AI use case?
It can be hard to get buy-in to develop your use case if you don’t prove the business value to all your relevant stakeholders at the outset. While they’ll vary by use case, we’ve compiled some helpful metrics you can use to project the value ahead of developing your proof of concept (PoC), as well as some important measures to keep track of once it’s up and running.
Pre-implementation AI metrics:
- Estimated AI model development costs - include the costs of data labelling, model training, testing etc.
- Estimated ongoing maintenance costs - the projected costs to update your AI model over time as more data is collected
- Time spent on current manual processes - Track hours specific roles spend on tasks that can be automated
- Error/rework rates of current processes - track errors or rework generated from manual tasks like data entry
- Customer satisfaction with current processes - conduct surveys to understand pain points your customers are experiencing with current processes
Post-implementation AI metrics:
- Actual AI model development and maintenance costs - track your spend against your estimates for to determine the variance
- Time savings from automated processes - track the hours saved by different job roles from reduced manual tasks
- Increased productivity - Track # of claims/orders processed, tasks completed by roles per unit/time
- Reduced error rates - track errors from automated tasks compared to manual performance as either a percentage or count
- Improved first contact resolution - track if AI helps resolve queries in initial contact vs multiple touches
- Decreased support costs - track reduced calls/emails to support teams in terms of both volume and/or cost savings
- Increased customer satisfaction - repeat surveys to measure any improvements in experience with your new automated processes
- Growth in relevant KPIs - track metrics like order value, customer lifetime value, churn rates over time, aligned to your business goals
What are the risks of not building an AI use case?
The temptation to rush head first into implementing the “latest and greatest” in AI tooling can be tempting, but you would be wise to pause for thought. While it might seem like the quickest route to value, there’s a lot at stake if it goes wrong. Here are the risk factors to consider if you’re tempted to skip over building a robust use case for AI.
The top 5 risks associated with failing to build a use case for AI:
(1) Missed revenue opportunities
Without a targeted use-case for AI, you’re likely to miss out on leveraging AI to tap into new revenue streams or enhance existing ones. For instance, AI can be used to personalise customer interactions and offers, leading to higher conversion rates and customer loyalty. Ignoring these opportunities can leave significant money on the table and allow your competitors who are more adept at leveraging AI to gain market share at your expense.
(2) Inefficiency and wasted investment
Investing in AI technology without a clear use-case often leads to underutilised resources. You could easily spend large amounts on advanced AI tools but see little return if these tools are not aligned with your specific business needs. This not only wastes precious financial resources but also can demoralise your teams, leading to lower productivity and innovation overall.
(3) Increased operational costs
AI can streamline operations and reduce costs by automating tasks, optimising processes, and improving decision-making. If you overlook the use of AI in these areas you’re going to continue to incur higher operational costs, such as infrastructure, team headcount, software licences, etc. compared to competitors who do employ AI effectively. This can affect the overall competitiveness and profitability of your business.
(4) Risk of technological obsolescence
AI technology evolves rapidly. If you don’t focus on the strategic implementation of AI you may find yourself stuck with obsolete technology that doesn’t serve your business needs. This not only requires further investment down the line to catch up, but also poses a risk of becoming irrelevant in a market where technological agility is crucial. A failure to keep up compounds quickly over time.
(5) Reduced customer satisfaction
Customers everywhere expect personalised, efficient, and innovative products and services. If you don’t use AI to enhance your customer experiences you might struggle to meet these expectations, leading to lower customer satisfaction and retention, presenting a real risk for your business.
Accelerating value for your business through AI
Here’s a recap of the key things you need to know about building an effective AI use case to deliver demonstrable value for your organisation, at pace:
- Start with clearly defining a specific problem statement and a desired quantifiable outcome. This will give you a “North Star” to guide your project.
- Focus on solving strategic business problems for the long-term, not just implementing technology for its own sake in the short-term. The focus should always be on business value, not on “shiny objects”.
- Think carefully about relevant quantitative and qualitative ways to measure ROI and business value over the long-term.